5 Ways F'inn is Using Generative AI
- Greg Robison
- Jun 9, 2024
- 7 min read
Updated: Dec 5, 2024
F'inn's usage of GenAI not only is saving us time but it's opening up new ideas and ways of working, we hear so much resistance focused only on the negative, but there's a lot of responsible and ethical use cases that are game changers. I truly feel bad for people who think it's worthwhile to miss this bus. - Brian Baecker
INTRODUCTION
At F’inn, an insights consultancy working with some of the most innovative companies in the world, we’re innately curious and have been deeply investigating Generative AI for the past few years. From the amazing pictures of novel faces generated by Generative Adversarial Networks (GANs) to the first time GPT-2 finished my sentence for me exactly as I would have, we’ve been working with the latest AI models to understand their capabilities and weaknesses, all while looking for specific applications than can benefit our business. Unlike previous software systems, Generative AI can produce original, previously unseen outputs, which is why it’s transforming our business by automating tasks, helping us find insights, and helping us work faster and smarter. We’ll walk through five examples of how F’inn is using GenAI every day to improve our work as experts in market research and innovation strategy.

1. Creating Bespoke Images for Proposals and Reports
At F’inn, we value the impact of visual content in our proposals and reports and Generative AI has become a key replacement for stale stock photos. By using diffusion models like Dall-e or Midjourney, we can generate custom images that are specifically tailored to our needs and preferences for each client. Either using online services or creating our own model, the process involves training the AI model on a huge dataset of relevant images, allowing it to learn the underlying patterns and styles. We then input key parameters, such as desired themes, color schemes, and composition, and the AI generates a range of unique images that align with these specifications.
Our materials are more engaging and memorable – with graphics perfectly tailored to each client’s brand identity and aesthetic preferences, we can leave a lasting impression. We also save time and costs compared to traditional methods of creating custom images from scratch. Our team can focus on higher-order needs like looking for insights about business strategy and not browsing Getty Images for something like might be relevant.
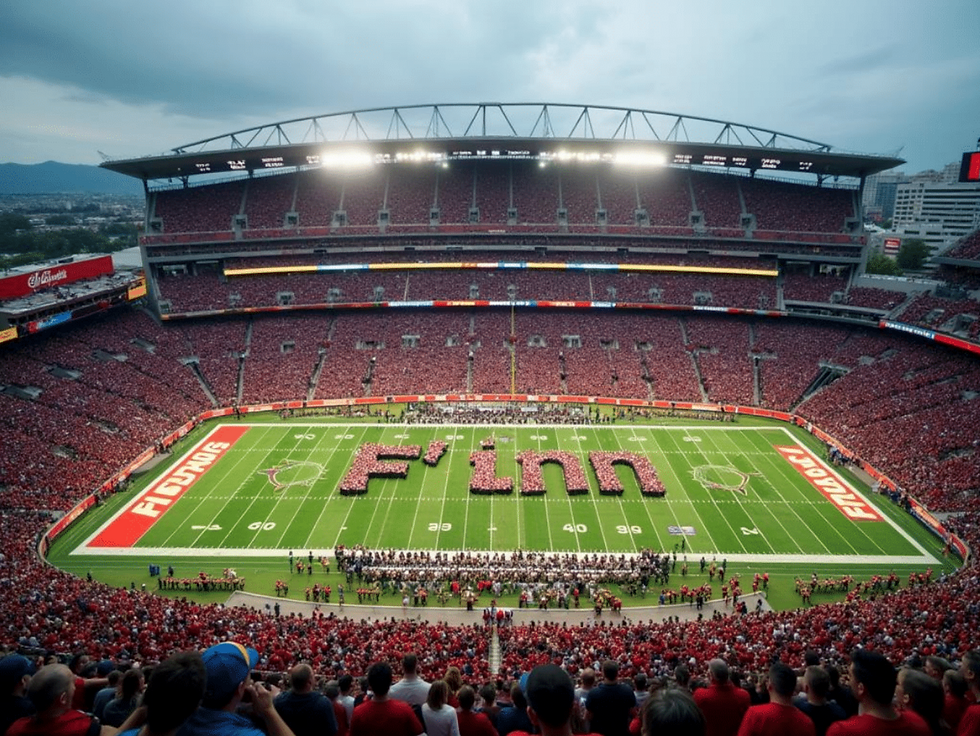
We can create images that relate to our passion projects like Mental Health or Sustainability. We can integrate our logo into materials in the same context in a way we never could. Our brand can transcend usual usages into any form we can think of.
2. Learning About New Categories and Businesses
Generative AI via Large Language Models (LLMs) like ChatGPT has become a time-saver for quickly learning about a new category that may be relevant for a new product or service. Previously, literature searches could take days to find the right sources and assimilate the information to something digestible. With LLM system’s ability to browse the web, they can find information and create summaries, pros/cons lists, comparison tables and more. The game changer for us is the ability to add references – we don’t have to worry about the AI hallucinating the information because we are given links to the information sources for us to verify and reference. With these tools we can gain a comprehensive understanding of unfamiliar industries and feel more confident identifying promising opportunities for growth and expansion.
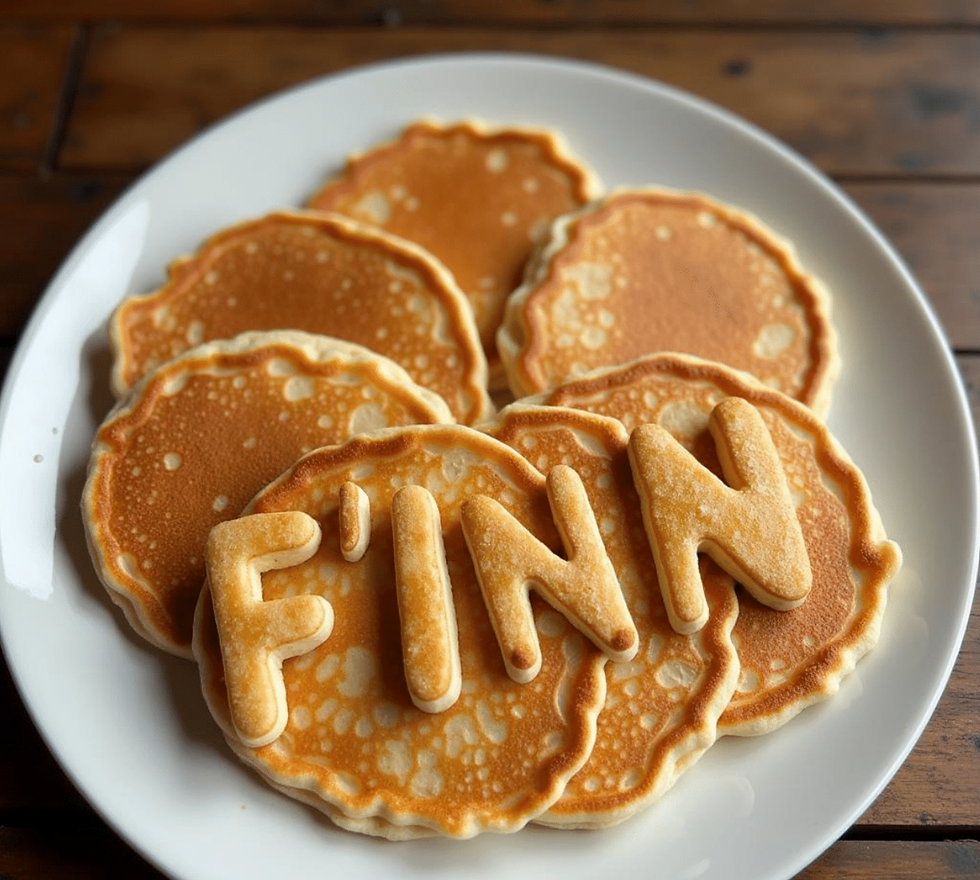
AI tools can bring diverse sets of data together, either by scraping relevant websites, social media platforms, news articles for up-to-date and accurate data on market trends, consumer preferences, and competitive activities. AI systems can then help us identify patterns and correlations within this data that would be more difficult manually (more on how we do this later). We can accelerate the research process, gathering information at a pace that would be impossible through traditional methods. With quickly-changing consumer sentiment, it’s especially important to be able to swiftly identify emerging trends and opportunities for quick adaptation in market. This depth of understanding is necessary when entering new markets or industries, and doing the necessary background research can mean the difference between success and failure in market.
3. Retrieval-Augmented Generation (RAG) for Transcripts and Survey Verbatims
In both our qualitative and quantitative survey research, we are often faced with huge and complex datasets, whether it’s 100 hour-long transcripts of in-depth discussions on the latest Augmented Reality products or thousands of open-ended responses as to why no one wants a drone flying around taking pictures of them. To help us look for themes, trends, and insights, we have been utilizing Retrieval-Augmented Generation (RAG) to handle large text data. RAG combines the strength of databases to efficiently search and locate relevant information with the generative capabilities of LLMs to produce coherent and contextually appropriate summaries and insights.
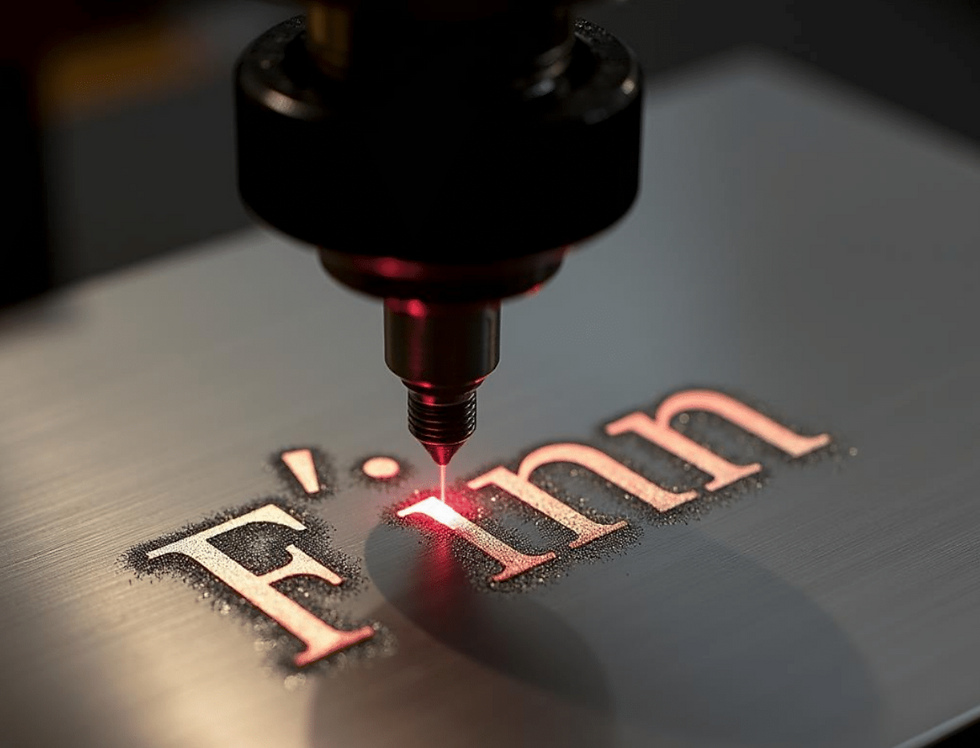
At F’inn, we have leveraged RAG to enhance our ability to process and analyze large amounts of interview transcripts in just a few hours – what used to take days of dedicated focus. We can automatically summarize key points, extract relevant insights, and identify recurring themes and sentiment to find insights. We’re not relying on LLMs to understand the topic – we’re providing them with the raw data to analyze, and the ability to cite references makes them less prone to making things up. We can quickly distill unstructured text into actionable intelligence. Being able to chat with your documents allows new ways to analyze data.
For transcripts and survey verbatims, we can quickly get to insights and then probe for depth to further develop the story. A good RAG system is only as good as its user at asking the right questions, smartly probing, and gut-checking responses for how well it lines up with other data points. We can process thousands of pages in minutes, allowing us to focus on higher-level analysis and strategy. We can create databases of reports and questionnaires, allowing our teams to search, filter, and retrieve specific information as needed using natural language. Did I mention you get references so you can look for yourself? In one study we were able to turn around highly actionable and specific insights on dozens of interviews just a few hours after completing them. With our expert interviewers and moderators, we can process and gain insights from more data much more quickly than ever.
4. Data Analysis via Python Coding
The other way F’inn is finding better insights in large datasets is by using GenAI’s ability to analyze data. If you’re doubting ChatGPT’s ability to do math, let alone something complex like a latent class factor analysis, you’re right – but what it is great at is coding in Python. It turns our natural language command of “do a latent-class factor analysis on this dataset” into Python code so it can run the analysis and then interpret the results for us. It has revolutionized the way I process, interpret, and derive insights from complex (and not so complex) datasets. As a so-so SPSS programmer, I’m no longer limited by my abilities and I can also automate repetitive things, like recoding data accurately. It is saving me time and lots of frustration.
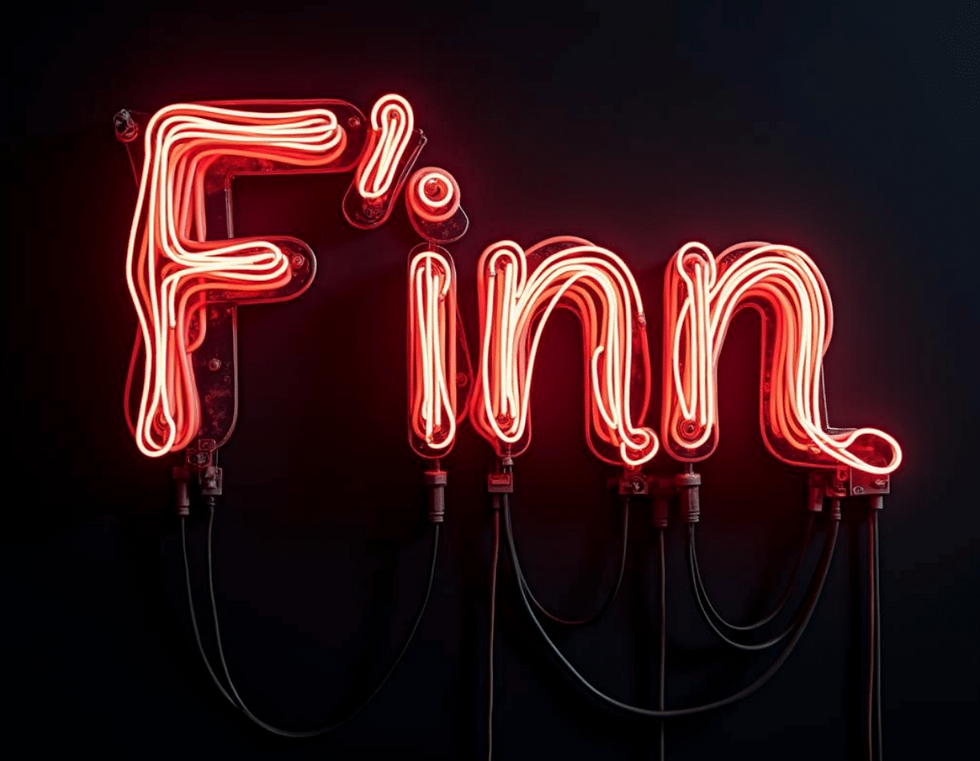
With faster data analysis and accurate results (you can watch the code being produced, run, with the resulting statistical output), we can now tackle complex analytical challenges that were previously beyond our reach in ability, time, and/or cost. For example, we have been able to develop predictive segmentations, key-driver analyses of purchase behavior, and logistic regressions for understanding complex relationships between demographic variables. We can also better forecast market trends and predict customer behavior with unprecedented precision. We have uncovered hidden patterns, relationships, and insights that would have gone unnoticed using more basic analytical techniques. This ability has given us a significant competitive edge, allowing us to make more informed decisions, optimize our strategies, and stay ahead of the curve in an increasingly data-driven business landscape.
5. Synthetic Qualitative and Quantitative Survey Respondents
One of the most interesting and close to my interests is in creating synthetic respondents using AI for qualitative and quantitative research that closely aligns with humans. We are using LLMs to generate realistic, diverse, and representative profiles of participants who can be used as virtual participants in surveys and focus groups. By grounding our synthetic respondents on real human data, transcripts, etc. we can create synthetic data that closely mimic characteristics and opinions of actual people. Chatting with synthetic personas on F’inn’s PersonafAI platform yields similar insights to talking with real people because of our expertise with both human consumer behavior and AI models.

We have started using these synthetic respondents to pretest our surveys or discussion guides – before we go talk with (presumably) real humans, let’s make sure the flow, probes, response lists, etc. all make sense. Especially when we are looking for low-incidence groups that are both expensive and hard to find, we can make sure we’ve tested everything with a few key target populations. It’s like taking a few swings from the on-deck circle before heading up to the plate to bat. We’re smarter, more efficient, and will have better data because of our practice.
With synthetic respondents in F’inn’s QuantifAI platform, they never get tired, you can conduct large-scale surveys that would be logically impossible with humans (e.g., evaluate 100 versions of this product and pick your top 5). We can create thousands of synthetic respondents, representing all ages, income levels, geographies, etc. all owing us to gather representative data on consumer preferences, brand perceptions, and even attitudes. We’ve also been using synthetic respondents to augment hard-to-reach populations, providing a way to gather detailed insights without the need for expensive and time-consuming recruitment. Whether it’s professional audio installers, personal investment advisors, or VR developers, F’inn’s synthetic respondents can reliably augment real humans to answer additional questions, provide color to quantitative results, or help refine hypotheses. All while removing much of the bias that comes from humans, such as social desirability bias, response bias, and lack of diversity, ensuring that our research results are more accurate, reliable, and representative.
CONCLUSION
At F’inn we embrace innovation and new technologies and have found many ways that GenAI is improving our work. From creating bespoke images for client proposals and reports to learning about new categories and businesses through AI-driven market research, this transformative technology has already revolutionized key aspects of our operations. We are reaping the benefits from applications of retrieval-augmented generation for transcripts and survey verbatims, the role of Python coding in automating and enhancing data analysis, and the groundbreaking concept of synthetic survey respondents. Each of these use cases demonstrates the potential of generative AI in streamlining processes, uncovering insights, and delivering expert results for our clients. By staying at the forefront of AI and other innovations, F’inn is continually exploring novel applications that we believe will benefit our clients through broader and deeper insights.
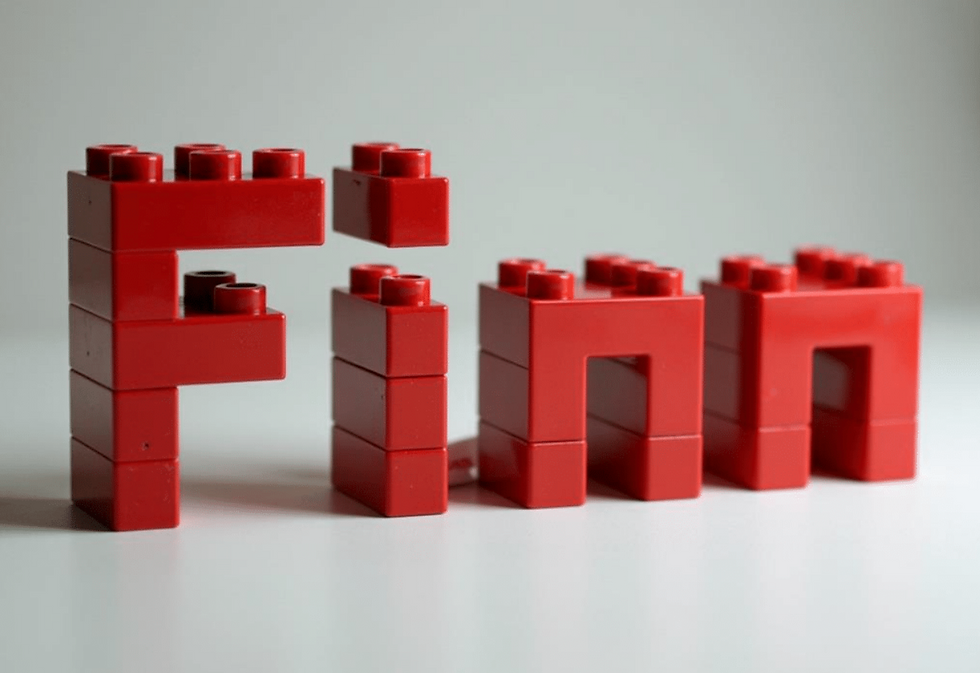